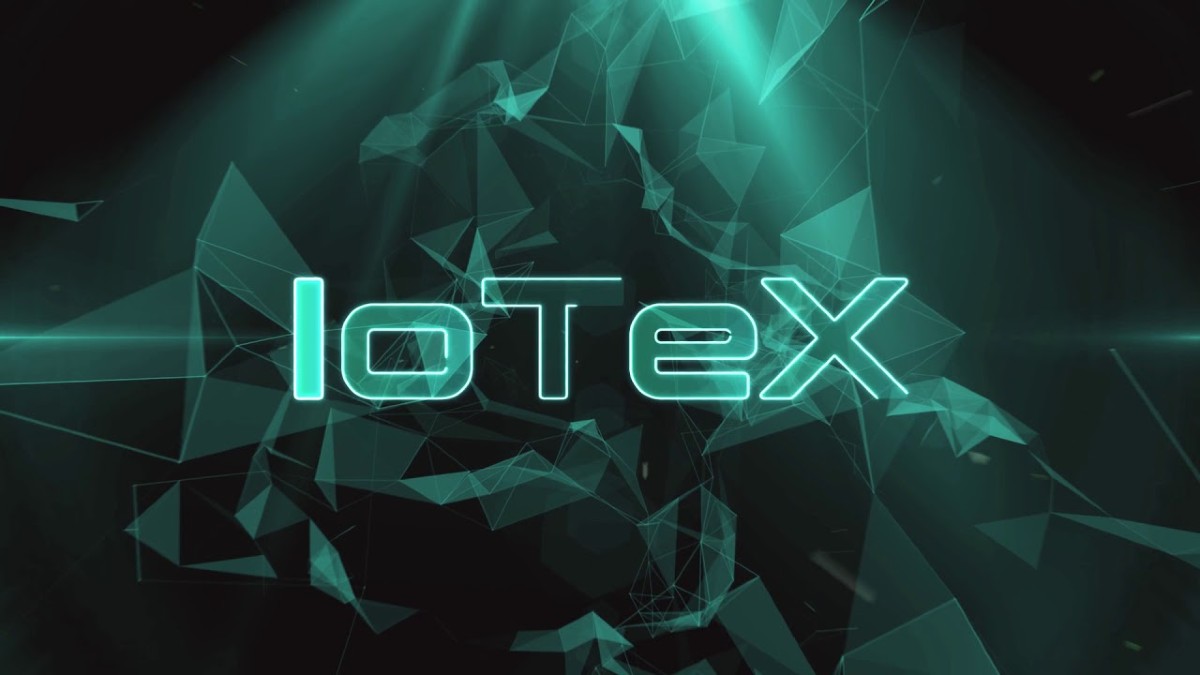
The Internet of Things industry is one of the most promising technological developments of our day.
By definition, the Internet of Things (IoT) is a network of physical and virtual objects that may interact via the Internet Protocol (IP).
Applications include B2C smart home systems and B2B logistics monitoring and energy grid control. Thus, IoT comprises hardware, software (called “platforms”), services, and connection.
In 2021, the global IoT market has reached $17.5 billion of total value.
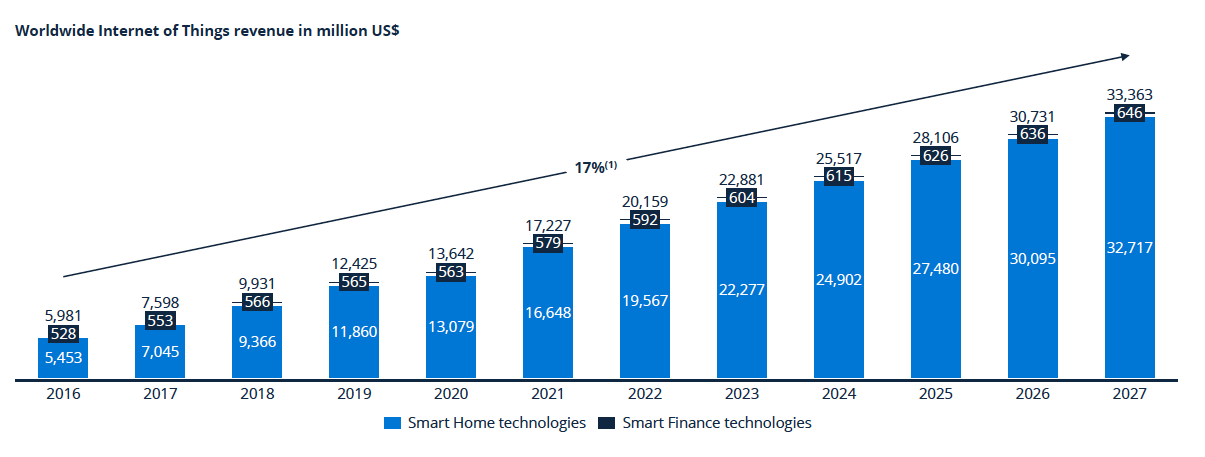
Consumers are rapidly using Smart Home technology, which accounts for 97% of global sales. Smart House technologies have the fastest growth compared to other categories, fueled by home upgrades during the COVID-19 epidemic.
The Internet of Things industry is diverse in terms of both application and brand. The IoT market is shaped by pure players (like PTC) as well as huge consolidated organizations with diverse goods and services.
The introduction of 5G communication standard is a game changer for speedy connectivity between IoT devices. Using edge computing instead of cloud computing speeds up procedures by collecting and analyzing data at the IoT device level.
Automation utilizing IoT also benefits industrial use cases, resulting in a new trend: the Industrial Internet of Things (IIoT). But as IoT use grows, so do cybersecurity dangers, as these devices become targets for hackers.
Blockchain has proven to have a significant impact on the Internet of Things by increasing safety and enabling the integration of more devices. The improvements in IoT device security speed up the adoption of this breakthrough invention and bring up new opportunities for businesses.
As of today, few IoT systems utilize the blockchain to transfer data. Blockchain technology allows for immutable and decentralized data transfer and both IoT and blockchain need conscious and non-intentional risk management.
For these reasons, blockchain technology can address several of the IoT cybersecurity needs, including integrity, secure communication, and resilience: it might bring additional security qualities like availability and accessibility to a secure micropayment system.
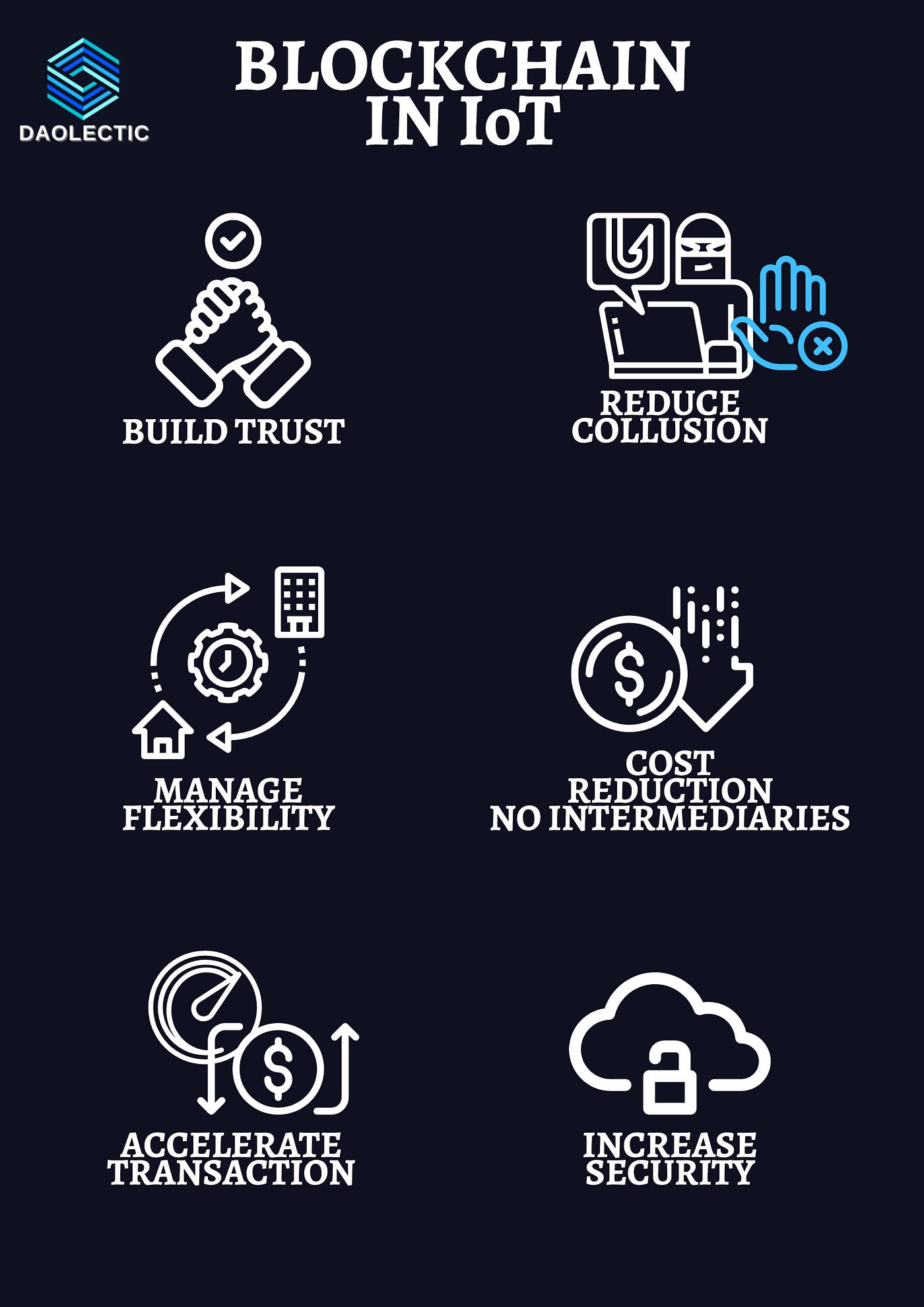
The ideal blockchain implementation in the IoT space must have no or minimal transaction costs, significant growth potential, and a scalable identity management procedure.
However, traditional blockchain does not address all IoT security concerns: personal data confidentiality and protection need additional encryption.
That’s where IoTeX stands out.
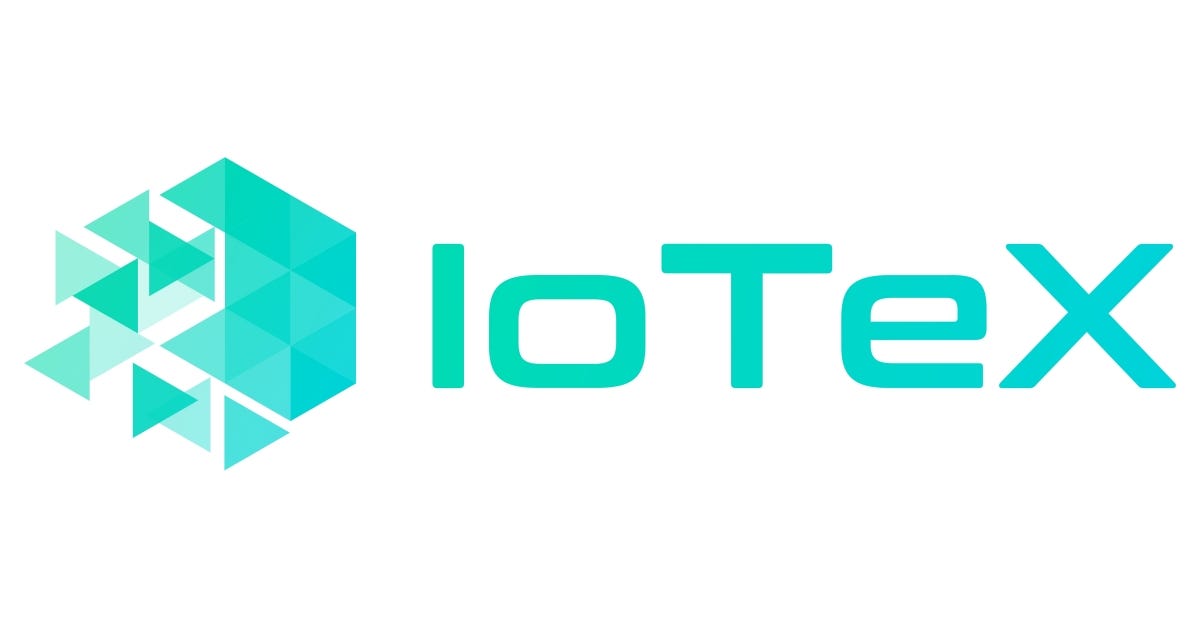
IoTeX
IoTeX was founded in 2017 by Raullen Chai, Qevan Guo, and Jing Sun. and deployed in February 2018.
IoTeX is a full-stack platform that enables trustworthy data from trusted devices to be used in trusted DApps.
It employs permissioned or permissionless blockchains, enhancing privacy with quick consensus and immediate finality.
IoTeX believes no one blockchain solution can meet all IoT needs. For this reason, they established specific platforms that will communicate with defined IoT devices, following the idea of separation of tasks.
Indeed, the specified level of IoT structures can only be managed by a certain level of blockchain complexity.
The IoTeX platform is composed of many technology layers:
- Roll-DPoS consensus with more than 60 decentralized delegates
- Secure Hardware: tamper-proof devices using Trusted Execution Environment (TEE) that work flawlessly with IoTeX
- Real-World Data Oracles: turn real-world events into verified data for IoTeX DApps
- Decentralized identity framework that allows users/devices to control their data and credentials
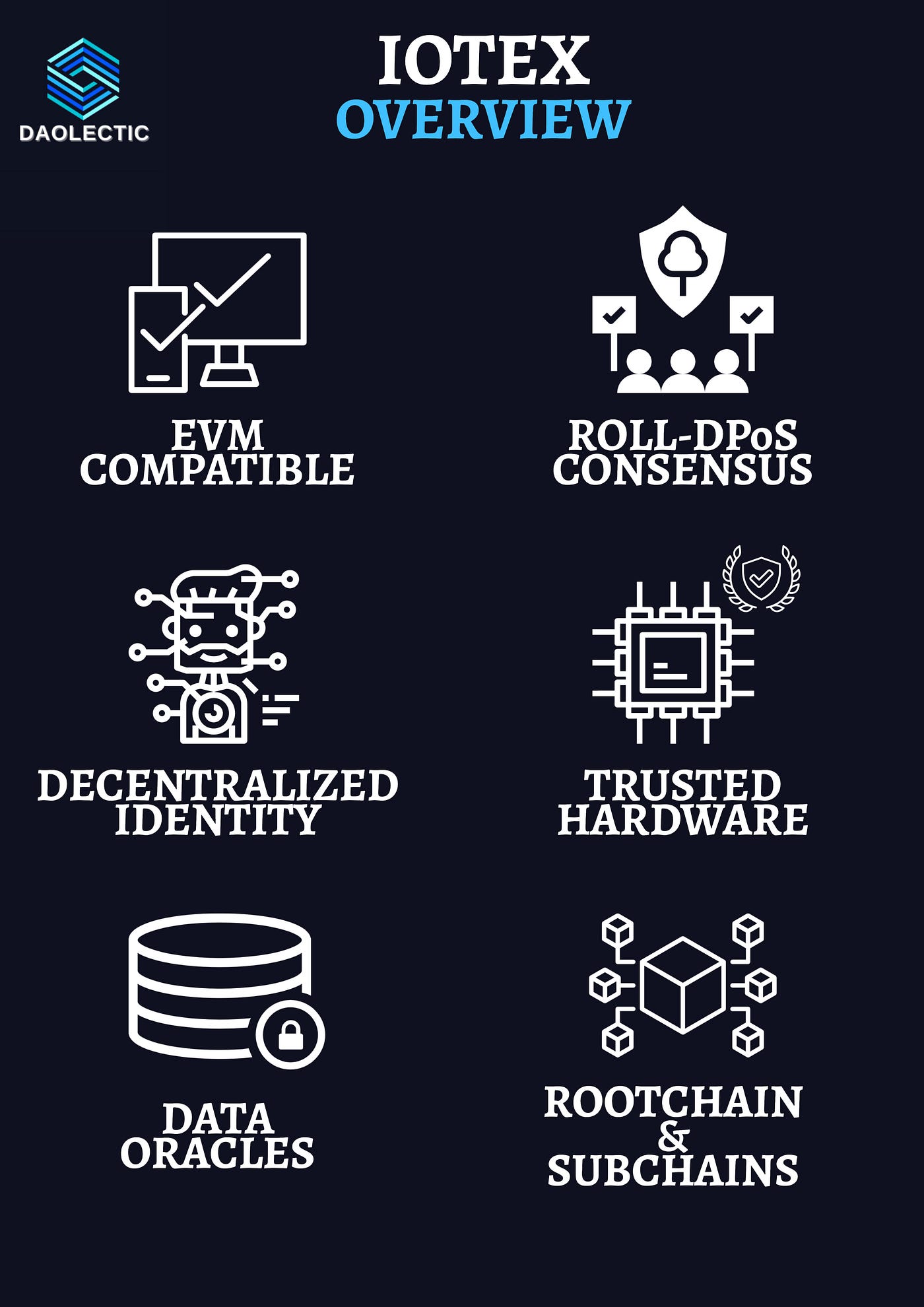
IoTeX Rootchain and Subchains — Fast Consensus with Instant Finality
IoTeX has a public permissionless root chain as well as many subchains.
Subchains may be permissioned or permissionless blockchains that allow smart contracts.
The root chain is a public blockchain that focuses on scalability, resilience, privacy-preserving functionalities, and subchain orchestration: it has been deployed to transmit value and data across subchains, supervise the different subchains along with settlement and anchor payments for them.
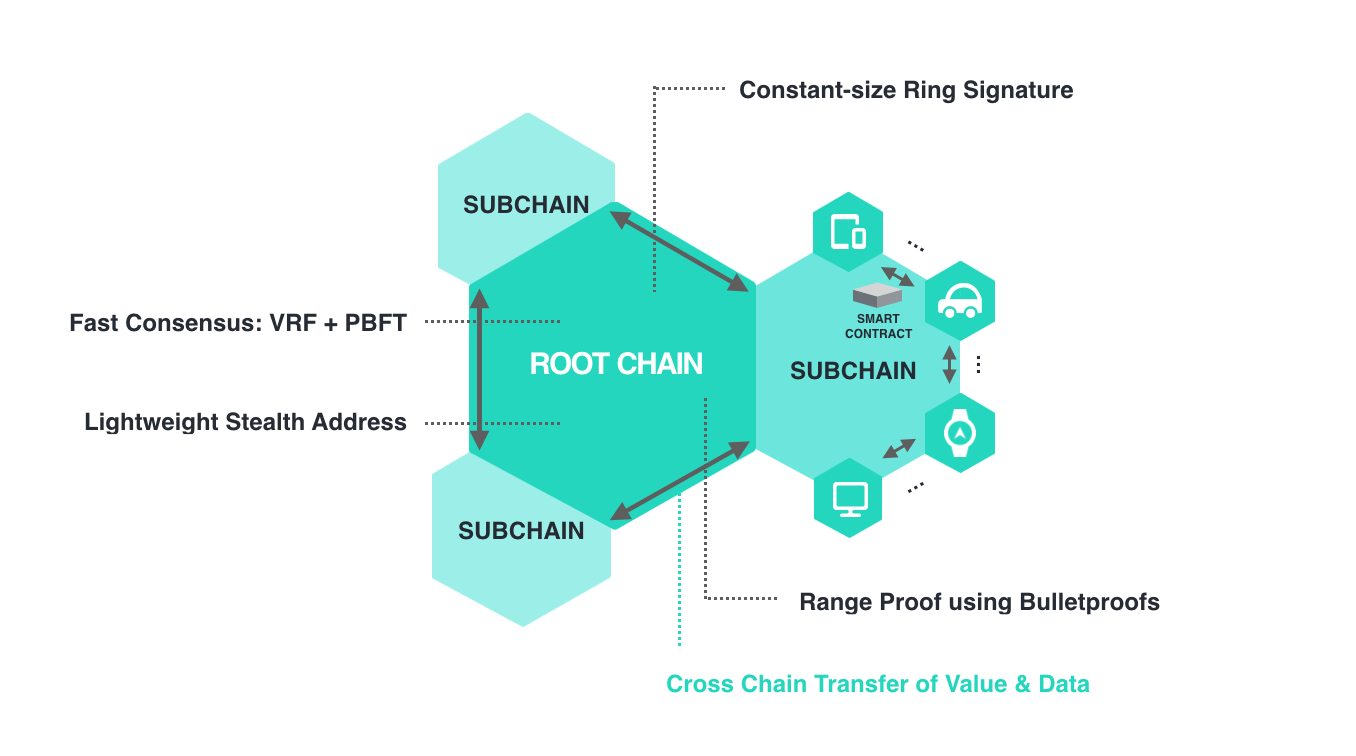
To ease transaction ordering, the IoTeX root chain employs the UTXO concept.
A subchain, on the other hand, is a blockchain that can be either private o public that uses the root chain to communicate with other subchains.
A subchain's key characteristics are flexibility and extensibility as they are needed to meet the diverse IoT applications. To function, a subchain is typically managed by operators with a strong stake in the root chain.
Additionally, the system lets users choose one or more delegated operators to act for them, with or without a bond. To seal new blocks, the delegator acts like a light client on the root chain, and like a full node on the subchain.
Consensus mechanism
IoTex root chain consensus delivers immutable blocks in real-time and it employs the so-called Roll-DPoS (randomized delegated proof of stake): token holders vote for their delegates, who are then ranked according to the number of votes they get.
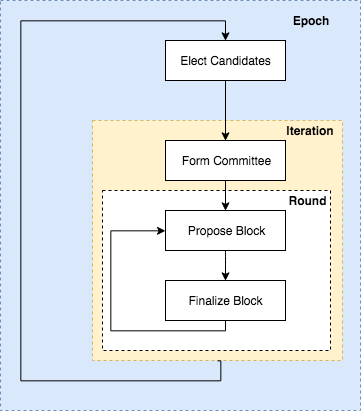
The delegates who received the most votes are known as the “consensus delegates” for the present epoch (1 hour). A randomization method then selects a sub-committee to preserve the agreement and generate new blocks for each new epoch.
Block finality is critical for IoTeX cross-blockchain communications. These interactions are based on simplified payment verification (SPV), a mechanism that allows a lightweight node to authenticate a transaction using a Merkle tree and block headers without downloading the complete blockchain. IoTeX employs two-way pegging (TWP) to allow token transfers to and from subchains.
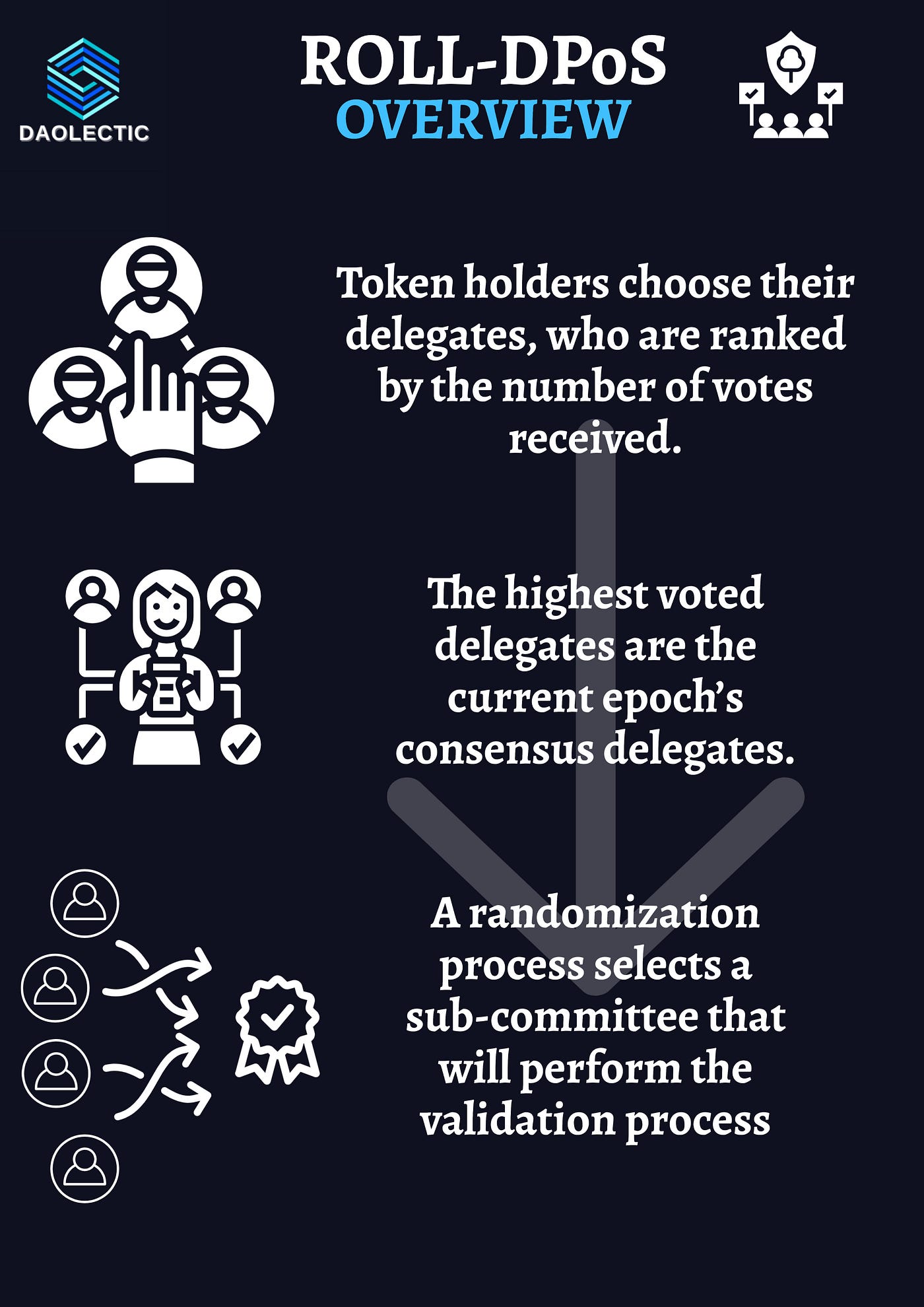
Secure Hardware
A core idea of the project is to have and provide final users with trusted devices for the data collection.
Hardware to be considered secured and tamper-proof needs to embed a Trusted Execution Environment, which are extremely secure and segregated enclaves that operate in parallel with a device’s/main machine’s system.
A TEE protects the confidentiality and integrity of all data and processes inside it.
IoTex’s goal is to make the first decentralized machines that can participate in the Internet of Trusted Things autonomously. In this regard, the company made the first hardware device that can’t be manipulated: the Pebble Tracker.
Pebble tracker
The Pebble Tracker has a TEE and a lot of sensors (GPS, climate, motion, and light) to get information from the real world and turn it into verifiable, blockchain-ready data. In addition to minting digital assets, smart contracts can be used to do things like train machine learning models and make crowdsourced climate indices bringing verifiable and trusted.
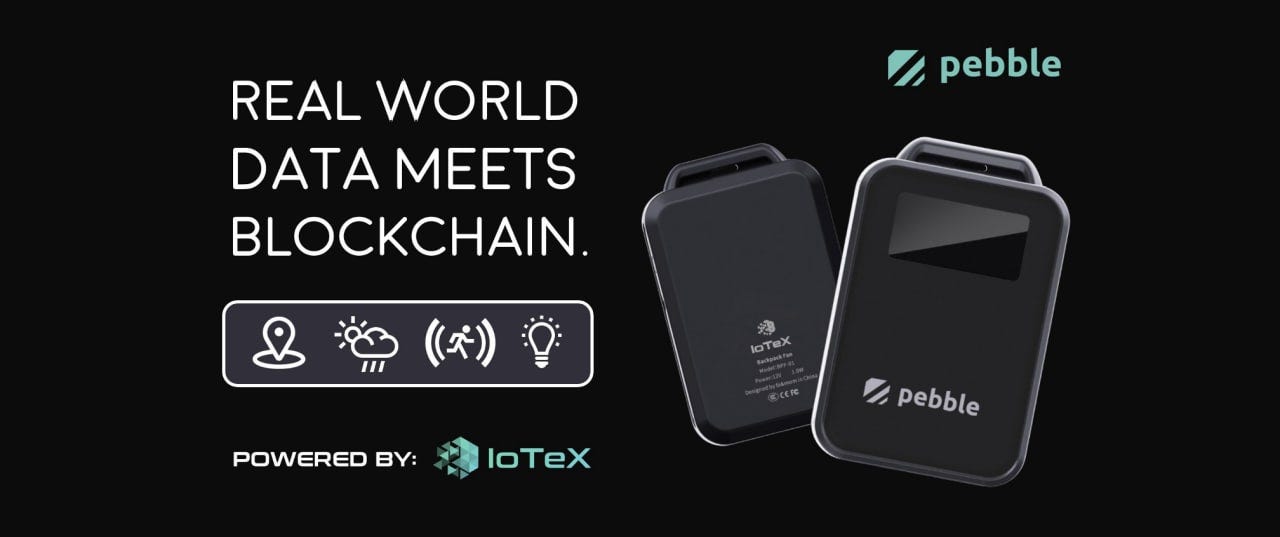
Decentralized Identity
Decentralized Identity (DID) is the “root of self-sovereignty” for the IoTeX platform. Unlike other blockchain networks, IoTeX has created a DID system for both individuals and machines. People and devices may interact directly using IoTeX since their IDs are interoperable and standardized. IoTeX DID also enables people and devices to own/control their data and identity over the IoTeX network.
The Industrial Internet Consortium is currently standardizing IoTeX’s DID technology and Identity & Access Management (IAM) architecture (IIC). It can link various application layers and enable user-centric data exchange across global IoT ecosystems with billions of IoT devices and millions of users.
Data oracles
Data oracles are required for smart contracts to access off-chain data. For the blockchain sector, IoTeX is constructing the world’s first data oracles that concentrate on verified real-world data from trustworthy devices, making IoTeX the first mover in this direction.
Real-world data on IoTeX will enable thousands of use cases and new on-chain assets supported by real-world data. As an approved data hub, IoTeX may now “serve” data to other blockchains like Ethereum and Polkadot.
Team
Raullen Chai, Qevan Guo, Xinxin Fan, and Jing Sun are the creators of IoTeX.
- In addition to co-founding IoTeX, Raullen Chai is a consultant at BootUP Ventures and a member of the Industrial Internet Consortium’s Industrial Distributed Ledger Task Group. He formerly served as Uber’s head of cryptocurrency research and development, as well as technical security.
- Qevan Guo is also one of Hyperconnect Lab’s co-founders. He worked for Facebook as a researcher and technical director.
- Xinxin Fan was a senior research engineer at the Bosch Research and Technology Center in North America prior to co-founding IoTeX. He also worked at the University of Waterloo as a research associate and project manager.
- Jing Sun serves as a managing partner at Sparkland Capital. She is a limited partner at Polychain Capital and a Rippling angel investor.
The whole IoTex team is made up of about 30 people including scientists, researchers, and numerous engineers from giants such as Google, Facebook, Uber, and Bosch.
Tokenomics
The $IOTX token enables the IoTeX blockchain. IOTX provides numerous utilities to facilitate trustworthy interactions amongst stakeholders, including users, Delegates (miners), application makers, and service providers.
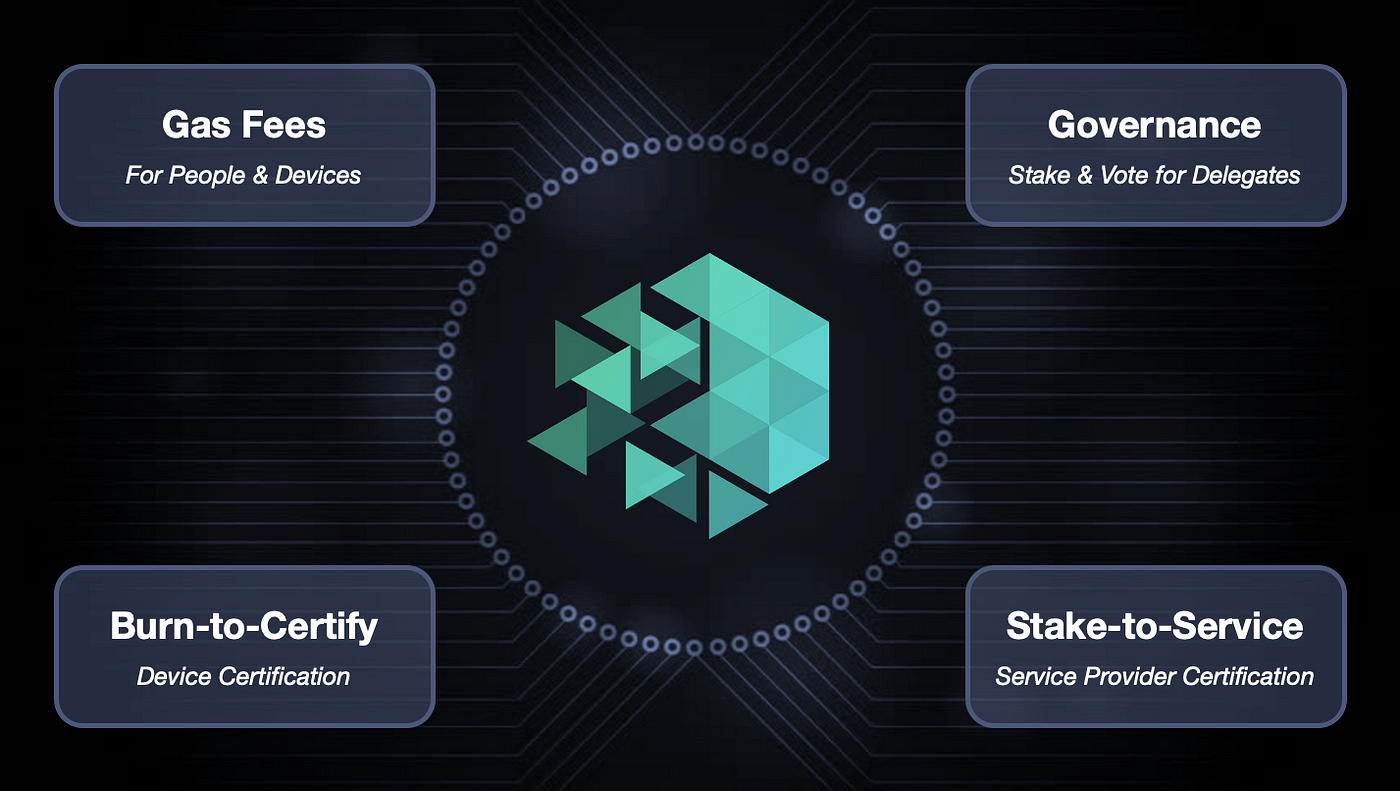
The IOTX token offers financial and reputational incentives to promote decentralized IoTeX Network governance/maintenance. Participants may spend, stake, or burn IOTX to access network resources. Increased demand and value of IOTX will encourage network members to maintain and extend the network.
Delegates stake IOTX to be eligible to participate in consensus, while service providers stake/spend IOTX to provide services to builders.
IOTX has a 10 billion maximum supply and is deflationary — IOTX is burnt for every new device and user registered to the IoTeX Network, rewarding long-term holders.
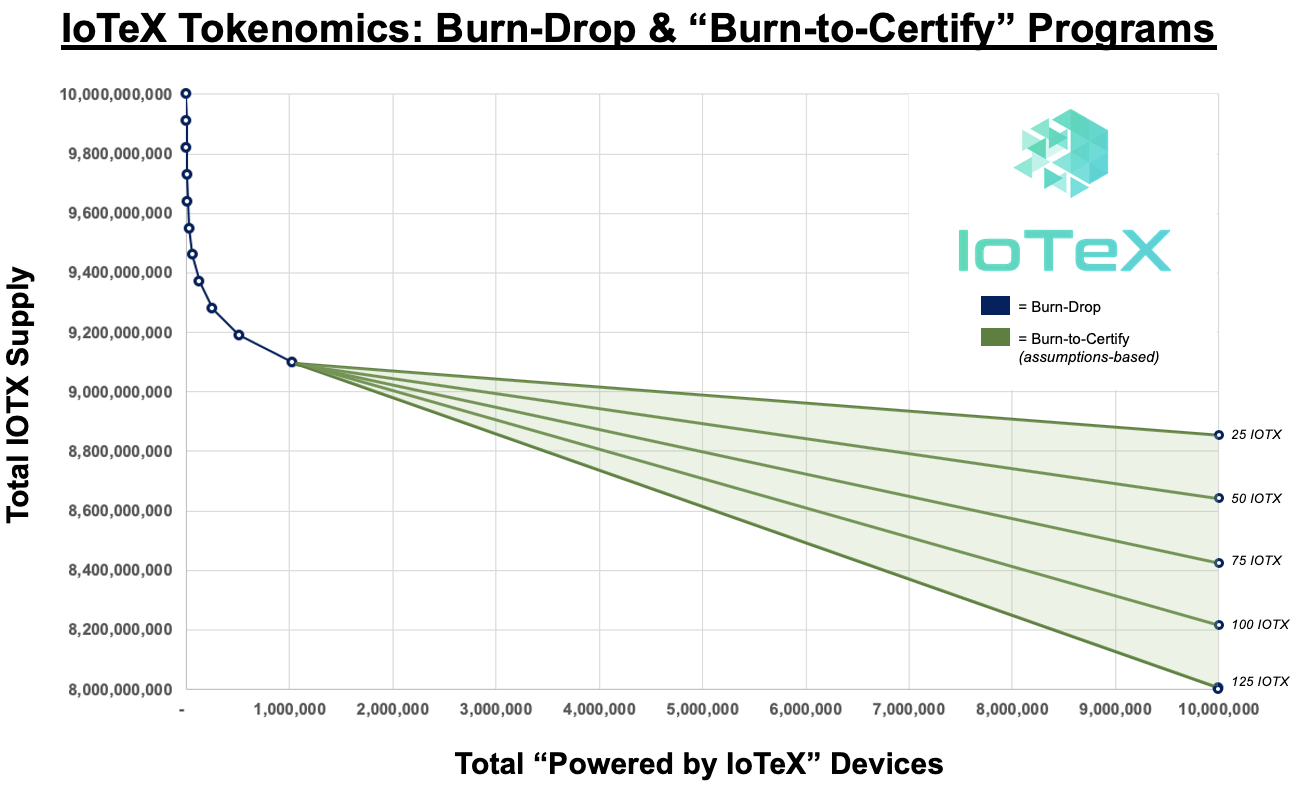
Following the onboarding of 1 million “Powered by IoTeX” devices, the “Burn-to-Certify” tokenomics will be enabled. Starting from that point on, builders will burn IOTX to access specific services/capabilities for each new device. As seen in the graph below, the overall supply of IOTX will drop with each additional “Powered by IoTeX” device.
Notably, these are the tokenomics that power the IoTeX blockchain, however, apps “Powered by IoTeX” may create their tokens and tokenomics based on their own incentives/rules.
- Maximum Supply: 10 Billion IOTX
- Total Supply: 8.8 Billion IOTX (after Burn-Drop)
- 900 Million IOTX (9% of max supply) will be gradually burned as 1 Million devices will be registered and confirmed on IoTeX
- 265 Million IOTX (2.65% of max supply) was burned in June 2020 as part of Mainnet GA activation
- Circulating Supply: 9.54 Billion IOTX
MachineFi
MachineFi is a concept used to describe the combination of blockchain and Internet of Things (IoT) technology.
This concept seeks to connect the physical world with the metaverse.
MachineFi also defines a network of smart devices that communicate with one another on the blockchain via the internet. On many fronts, blockchain has developed a robust framework for enabling decentralization.
IoTeX 2.0 intends to decentralize the MachineFi sector, allowing smart device users to engage in a rising trillion-dollar economy free of the constraints imposed by centralized data providers.
The “Proof-of-Anything” idea will be launched in the MachineFi upgrade. This will let IoT devices provide on-chain proofs of real-world events such as health measurements and GPS positions.
Conclusions
Blockchain technology can meet several cybersecurity requirements for IoT devices because it is distributed and can’t be changed.
However, a single blockchain implementation that doesn’t have other ways to deal with complexity, like smart contracts, edge, and cloud computing, can’t meet all of the security requirements that IoT platforms need to meet.
Identity and Access Management is an important part of building a strong defense against intentional risks that IoTex decided to tackle from day 1.
Disclaimer
This is not, in any case, financial advice, the goal of my research will always be to dive deep into projects and study them from different angles, I do include personal opinions based on my experience with similar projects that I have recently studied.
I am and will always be open to discussion.
Follow us on Twitter:
@daolectic
Please always do your research before investing in anything.
You must be logged in to post a comment.