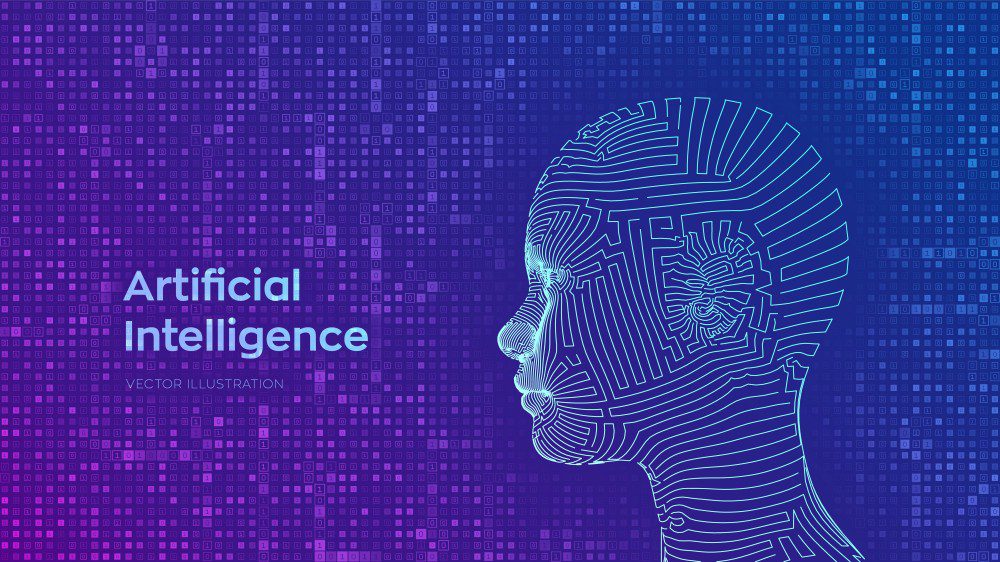
Analysts predict an AI boom, driven by possibilities and record funding. While challenges remain, a hybrid approach combining the best of the realm may finally send it sailing into the mainstream.
Artificial intelligence (AI) is becoming the dominant trend in data ecosystems around the world, and by all counts, it will accelerate as the decade unfolds. The more the data community learns about AI and what it can do, the faster it empowers IT systems and structures. This is primarily why IDC predicts the market to top $500 billion as early as 2024, with penetration across virtually all industries driving a wealth of applications and services designed to make work more effective. In fact, CB Insights Research reported that at the close of Q3 2021, funding for AI companies had already surpassed 2020 levels by roughly 55%, setting a global record for the fourth consecutive quarter.
In 2022, we can expect AI to become better in solving practical problems that hamper unstructured language data-driven processes, thanks to improvements in complex cognitive tasks such as natural language understanding (NLU). At the same time, there will be increased scrutiny into how and why AI does what it does, such as ongoing efforts by the U.S. National Institutes of Standards and Technology (NIST) aimed at more explainable AI. This will require greater transparency into AI’s algorithmic functions without diminishing its performance or raising costs.
You shall know a word by the company it keeps
Of all the challenges that AI must cope with, understanding language is one of the toughest. While most AI solutions can crunch massive volumes of raw numbers or structured data in the blink of an eye, the multitude of meanings and nuances in language, based on the context they are in is another matter entirely. More often than not, words are contextual, which means they convey different understandings in different circumstances. Something easy and natural for our brains is not that easy for any piece of software.
This is why the development of software that can interpret language correctly and reliably has become a critical factor in the development of AI across the board. Achieving this level of computational prowess would literally unleash the floodgates of AI development by allowing it to access and ingest virtually any kind of knowledge.
NLU is a vital piece of this puzzle by virtue of its ability to leverage the wealth of language-based information. Language inhabits all aspects of enterprise activity, which means that an AI approach cannot be complete without extracting as much value as possible from this type of data.
A knowledge-based, or symbolic AI approach, leverages a knowledge graph which is an open box. Its structure is created by humans and is understood to represent the real world where concepts are defined and related to each other by semantic relationships. Thanks to knowledge graphs and NLU algorithms, you can read and learn from any text, out-of-the-box, and gain a true understanding of how data is being interpreted and conclusions are being drawn from that interpretation. This is similar to how we as humans are able to create our own specific, domain-oriented knowledge, and it enables AI projects to link its algorithmic results to explicit representations of knowledge.
In 2022, we should see a definitive shift toward this kind of AI approach combining both different techniques. Hybrid AI leverages different techniques to improve overall results and better tackle complex cognitive problems. Hybrid AI is an increasingly popular approach for NLU and natural language processing (NLP). Bringing together the best of AI-based knowledge or symbolic AI and learning models (machine learning, ML) is the most effective way to unlock the value of unstructured language data with the accuracy, speed and scale required by today’s businesses.
Not only will the use of knowledge, symbolic reasoning and semantic understanding produce more accurate results and a more efficient, effective AI environment, it will also reduce the need for cumbersome and resource-intensive training, based on wasteful volumes of documents on expensive, high-speed data infrastructure. Domain-specific knowledge can be added through subject matter experts and/or machine learning algorithms leveraging the analysis of small and pinpointed training sets of data to produce highly accurate, actionable results quickly and efficiently.
The world of hybrid AI
But why is this transition happening now? Why hasn’t AI been able to harness language-based knowledge previously? We have been led to believe that learning approaches can solve any of our problems. In some cases, they can, but just because ML does well with certain needs and specific contexts doesn’t mean it is always the best method. And we see this all too often when it comes to the ability to understand and process language. Only in the past few years have we seen significant advancements in NLU based on hybrid (or composite) AI approaches.
Rather than throwing one form of AI, with its limited set of tools, at a problem, we can now utilize multiple, different approaches. Each can target the problem from a different angle, using different models, to evaluate and solve the issue in a multi-contextual way. And since each of these techniques can be evaluated independently of one another, it becomes easier to determine which ones deliver the most optimal outcomes.
With the enterprise already having gotten a taste of what AI can do, this hybrid approach is poised to become a strategic initiative in 2022. It produces significant time and cost benefits, while boosting the speed, accuracy and efficiency of analytical and operational processes. To take just one example, the process of annotation is currently performed by select experts, in large part due to the difficulty and expense of training. By combining the proper knowledge repositories and graphs, however, the training can be vastly simplified so that the process itself can be democratized among the knowledge workforce.
More to Come
Of course, research in all forms of AI is ongoing. But we will see particular focus on expanding the knowledge graph and automating ML and other techniques because enterprises are under constant pressure to leverage vast amounts of data quickly and at low cost.
As the year unfolds, we will see steady improvements in the way organizations apply these hybrid models to some of their most core processes. Business automation in the form of email management and search is already in sight. The current keyword-based search approach, for instance, is inherently incapable of absorbing and interpreting entire documents, which is why they can only extract basic, largely non-contextual information. Likewise, automation email management systems can rarely penetrate meaning beyond simple product names and other points of information. In the end, users are left to sort through a long list of hits trying to find the salient pieces of knowledge. This slows down processes, delays decision-making and ultimately hampers productivity and revenue.
Empowering NLU tools with symbolic comprehension under a hybrid framework will give all knowledge-based organizations the ability to mimic the human ability to comprehend entire documents across their intelligent, automated processes.
By Marco Varone, CTO at expert.ai on March 2, 2022 in Artificial Intelligence